Provable accuracy advantage in machine- learned quantum models of classical stochastic processes
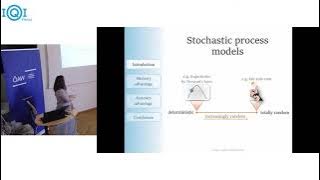
However, highly complex processes require a vast amount of memory for simulation. If the amount of available memory is limited, this can lead to inaccuracies in the simulation.
It has previously been shown that quantum information processors can reduce the memory required to accomplish exact simulation. Thereby, stochastic process simulation has been established as a new application that can benefit from quantum resources. However, until now, quantum works in stochastic process simulation assumed knowledge about the process to be modelled and considered only exact simulation. This can be unrealistic, particularly in noisy real-world scenarios.
Here, we show how quantum information processing provides an advantage in accuracy, given constrained memory resources. We introduce a learning algorithm, which discovers dimensionally reduced quantum models that attain a higher accuracy than their best classical counterparts. Our algorithm directly uses the output data of a stochastic process, instead of requiring knowledge about the underlying model. The results represent one of the few cases in quantum machine learning where the quantum advantage is rigorously proven.
Kommentare (0)
Keine Kommentare gefunden!